题目:Bayesian regression models accounting for unidirectional misclassification
嘉宾:夏超雄博士(Northern Illinois University)
地点:管理科研楼EMBA第五教室
时间:2016年6月17日(周五)下午3:30
主办:管理学院统计与金融系、安徽省金融信息重点实验室
摘要: In this talk, we consider unidirectional misclassification, meaning that the direction of classification error is known. First, we investigate the identifiability of Bayesian regression models when the variable subject to unidirectional misclassification is a covariate. In the Bayesian framework, we consider whether knowledge of the direction of error suffices, so that adjustment for misclassification can be undertaken without any source of information on the magnitude of error. While measurement error models are generally nonidentified without such information, for the case of unidirectional misclassification, we do obtain model identifiability when the response variable is non-binary. For the binary response model that is nonidentified, we examine the extent of partial identification. The limiting posterior distributions of the parameters are obtained for this partially identified model, for two different prior distributions. The second case we study is when unidirectional misclassification happens in a response variable. We obtain theoretical results on the identification of the logistic and Poisson regression models. Misrepresentation in insurance is a special case of unidirectional misclassification that is of particular concern.
Based on the identifiability we obtained from the earlier case, we propose to embed the predictive analysis of misrepresentation risk by specifying a binomial regression model on the misrepresentation probability. The last proposed method allows for multiple factors that are subject to misrepresentation, while accounting for other correctly measured rating factors. We demonstrate the identifiability of all the models through both theoretical and simulation studies. Case studies are performed using Medical Expenditure Panel Survey data.
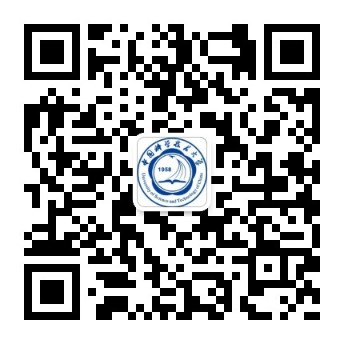
微信扫码关注
中国科学技术大学管理学院微信公众号